AI: Revolutionising the UK's Logistics Industry
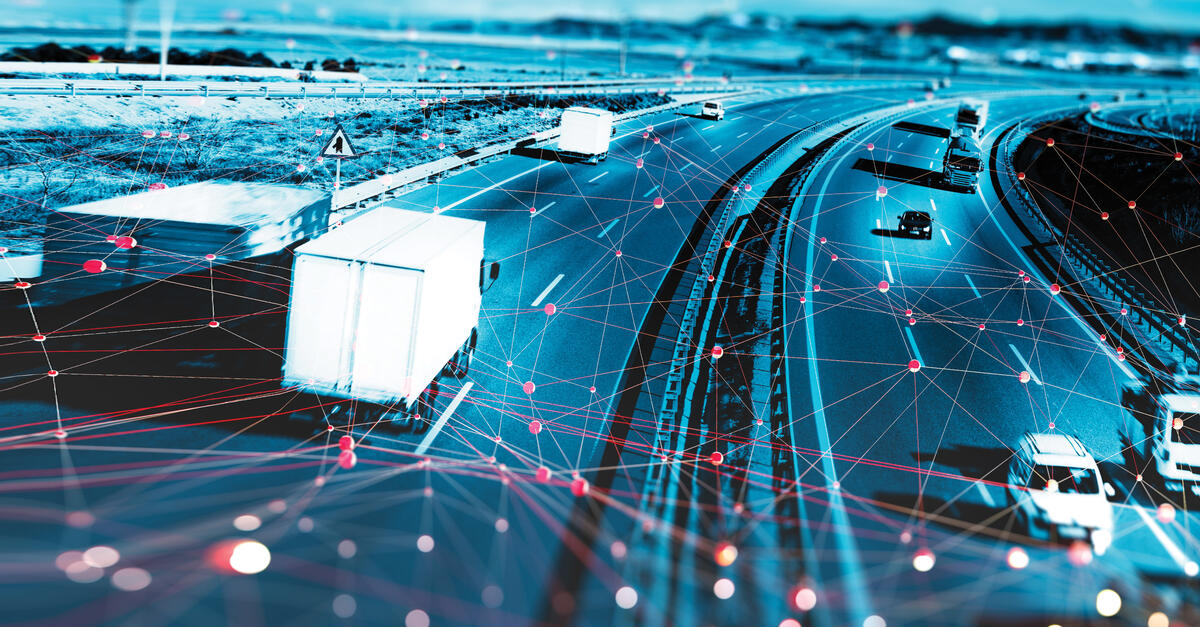
Artificial intelligence (AI) is transforming the logistics and supply chain landscape, creating unprecedented opportunities for businesses. However, many organisations remain unsure about how to effectively implement AI in their operations.
"AI is a constantly evolving field," said Chris Caplice, Executive Director of the MIT Centre for Transportation and Logistics. "It's not static; it's aspirational. What was considered AI 30 or even 20 years ago is no longer considered cutting-edge. It's always pushing the boundaries of what we think is possible."
When considering AI implementation, managers must understand the interplay between different analytical approaches, including traditional AI, generative AI, and operations research, according to Caplice, who spoke at a recent webinar hosted by MIT Sloan Management Review.
Caplice and Lior Ron, Founder and CEO of Uber Freight, delved into the analytical tools employed in supply chain management, the logistical challenges AI can address, and the broader managerial benefits of AI integration in the logistics sector.
Unpacking the Analytical Toolkit
Caplice highlights the importance of understanding the evolution of AI in logistics within the context of existing tools. Traditional AI focuses on analysing data to complete specific tasks. Generative AI, on the other hand, utilises large language models to extract context, summarise information, and create new content. Operations research employs scientific methods to study systems involving human decision-making, utilising techniques like linear programming and network models.
Caplice stresses that these methods are complementary and not mutually exclusive. Operations research combined with AI can be particularly effective in numerous applications.
Tackling Logistics Challenges with AI
Ron outlines several pressing logistics challenges that AI technologies are well-positioned to address:
Fragmented supply chains: AI can help connect and optimise fragmented networks to drive better outcomes.
Market volatility: AI can help navigate price fluctuations and service disruptions.
Safety concerns: AI can contribute to addressing safety issues related to COVID-19, driver safety, and digital fraud.
Environmental impact: AI can help mitigate the environmental impact of trucking and freight on climate change.
Innovative solutions are emerging to address these challenges. Uber Freight, for instance, has leveraged machine learning to pioneer algorithmic carrier pricing. This ensures carriers receive guaranteed upfront pricing for trucking and freight. "By analysing hundreds of different parameters, we've been able to create a model accurate enough to introduce a marketplace free from the friction, guesswork, and back-and-forth negotiations inherent in traditional price estimation," Ron explained.
Uber Freight is also employing machine learning to optimise vehicle routing, a complex problem involving finding the most efficient route for deliveries. Trucks in the UK often travel with a significant portion of their capacity empty, wasting time, fuel, and contributing to unnecessary carbon emissions. By algorithmically designing optimal routes, the company has managed to reduce empty miles to between 10% and 15%.
A Multifaceted Approach to Routing
While Uber Freight is a prominent example of AI application in vehicle routing, smaller businesses typically rely on operations research and human problem-solving to minimise transportation time, cost, and distance. However, for larger, more complex routes, traditional methods fall short.
The MIT Intelligent Logistics Systems Lab is addressing this challenge by combining traditional AI, generative AI, and operations research to enhance routing outcomes. "Initially, AI plays a supporting role, but as we progress, it's assuming a more central role," Caplice observed. "Ultimately, we aim for generative AI to take the lead in solving increasingly complex aspects of the problem."
Managerial Benefits of Generative AI in Logistics
Caplice highlights the limitations of using traditional operations research approaches in logistics. Every time new complications arise â such as varying time windows, street sizes, or truck capacities â algorithms need to be adjusted. Generative AI offers a more generalised solution, eliminating the need for constant algorithm tweaking.
This leads to significant performance improvements in solving larger logistical problems. Beyond this, generative AI offers additional managerial benefits:
Superior performance: AI models consistently outperform their training data, meaning they perform better on new, unseen data than on the data used during training. This saves time and effort as organisations don't need to meticulously vet routes beforehand.
Continuous learning: AI models can continuously learn and adapt to changing routing policies. If a policy shifts, the model will automatically update itself, eliminating the need for manual algorithm adjustments.
Generalisability: AI models are not constrained by specific problem sizes or characteristics. They can effectively adapt to varying parameters like vehicle capacities.
Unprecedented problem-solving: AI models excel at generalising solutions to previously unseen problems.
"Machine learning, AI, and generative AI are taking a large language model approach to solving what has been effectively addressed by operations research, but doing it faster, more comprehensively, and solving for objectives that go beyond the traditional," Caplice remarked. "We see significant opportunity here, and the research continues to evolve."
The integration of AI in logistics is revolutionising the industry. By harnessing the power of AI, businesses can optimise their operations, enhance efficiency, and navigate the complexities of the modern supply chain, ultimately delivering tangible benefits to both themselves and their customers.